The technical feasibility versus the economics of Artificial Intelligence
US-based Martin Fleming, Research Associate at The Productivity Institute, discusses the economics behind Artificial Intelligence
Artificial intelligence (AI) services have generated global attention. Offerings such as OpenAI’s ChatGPT are fascinating business leaders and the public alike while at the same time raising renewed fears that workers can be replaced in a wide range of occupations. But there is a need to recognise the gap between the technical feasibility of AI automation and the economics of AI investment. AI automation analysis focuses on which tasks will be automated – independent of cost. However, AI automation requires massive computer power (“computing”), data, and skilled worker expense.
For all the excitement, the emergent capability of AI has raised a classic question in strategy and operations research. Should businesses make their own AI systems or buy a third-party service? Recent work has shown that if firms buy AI-as-a-Service, paying a monthly fee as opposed to making their own very expensive solutions, the available service obviates the need for massive investment. Consequently, business leaders are asking whether firms should wait, postpone investment and, when available, buy a third-party service.
Clarifying AI investment decisions, informs broader questions of where AI automation will happen most quickly and hence what types of public policies will be important to avoid structural unemployment, maximise firm productivity, and possibly improve aggregate economic and productivity growth.
Large Language Models (LLMs)
Estimates of employment and labour market impacts of AI are already beginning to appear. Research has found it is technically feasible for approximately 80% of the US workforce to have at least 10% of their occupational tasks affected by the introduction of LLMs and that approximately 19% of workers might see at least 50% of their tasks impacted. The authors also find that, with access to LLMs, it is feasible that about 10% of tasks currently performed by US workers could be completed by LLMs significantly faster and at the same level of quality with high income occupations realising greater exposure.
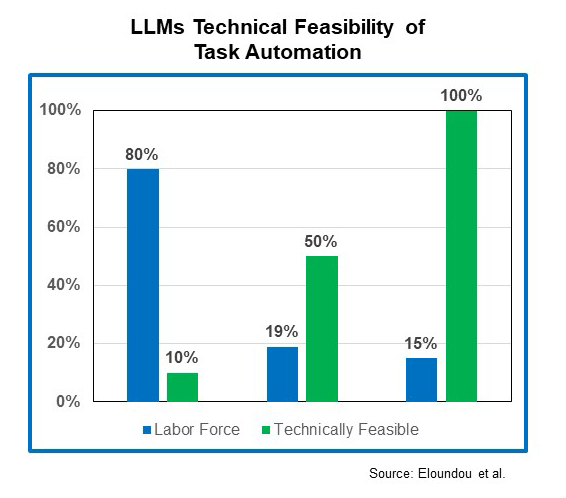
LLM’s Technical Feasibility of Task Automation
However, the nascent state of LLMs limits the availability of data about the development, training, and inference costs. Consequently, the economics of model deployment, diffusion, and the new business models they might spawn, are largely unknown with their economic feasibility remaining in doubt.
Computer vision models
By contrast, computer vision models have a much longer history, providing fertile ground for understanding the economics of AI. Computer vision is a field of AI that uses machine learning and neural networks to teach computers and systems to derive meaningful information from digital images, videos and other visual inputs—and to make recommendations or take actions when they see defects or issues.
In the 1960s, when AI emerged as a field of research and study, the quest to encode images and solve vision problems began. Today, vision applications are widespread, for example, in healthcare and manufacturing. Acemoglu and Restrepo report a fourfold increase in industrial robots in the US and western Europe between 1993 and 2007, many with vision capabilities. Similarly, recent progress has been reported in the development of healthcare-related computer vision applications, for medical imaging, video, and clinical deployment.
Not only are computer vision models relatively mature, but image data is freely available. ImageNet is a large visual database consisting of more than 14 million images, hand-annotated to indicate pictured objects. ImageNet contains more than 20,000 categories, with a typical category consisting of several hundred images.
Similar to many AI models, to achieve the required level of accuracy, computer vision cost is driven by computing, data, and technical human skill. For applications, ImageNet data are available on an open-source basis, largely at zero cost. However, if model performance improvement is required, the addition of labelled data can increase data cost to 70% – 90% of building and running costs. Training cost – substantial in most cases — is fixed for required accuracy and entropy, but the data costs are not.
Given that the cost per datapoint varies across use cases and acquisition methods, data costs will be a significant determinant in the proliferation of computer vision models. The cost of data can range from zero, where the data is already collected and labelled, to very high when highly skilled labour is required. The running costs are vanishingly small. With such massive upfront investment, cost minimisation requires deployment at scale.
Comparing the relative costs of AI with existing labour costs is the important ratio. The investment cost data for fine-tuning computer vision systems is compared to the wages for the occupational tasks relevant for vision function. Unlike the cost of computer vision, labour compensation does not exhibit obvious economies of scale. Consequently, while 80% of vision tasks are automatable, at current costs, only 18% of at-risk vision tasks in the US private, nonfarm business sector would be automated at the firm level. Only in limited cases are the marginal benefits large enough to justify the development and operations costs of such systems.
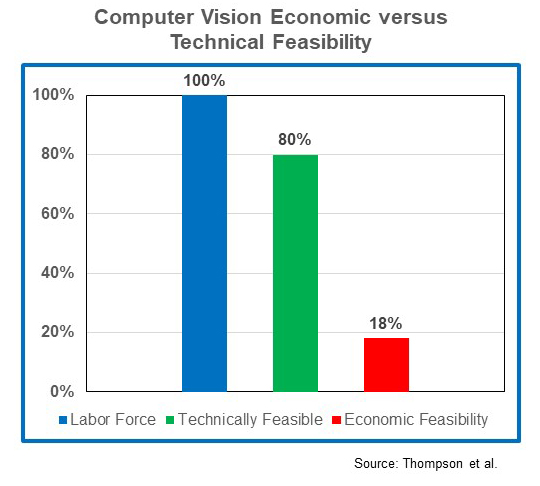
Computer vision – Economic vs. technical feasibility
AI as a service
The creation of platforms that offer AI-as-a-service could achieve the scale required for marginal benefits to exceed marginal costs. The share of tasks that are cost-effective to automate increases with the creation of platforms that offer AI-as-a-service. However, the extent of the future emergence of an AI-as-a-service platform industry remains unknown. With business model innovation and broad development across industries and business processes, automatable vision tasks could exceed 80% of the tasks automatable by computer vision AI applications. However, such an outcome remains highly speculative.
The impact of AI extends beyond the scope of computer vision. Nonetheless, insights from the economics of computer visions models will be helpful as LLMs proliferate. AI systems that rely on foundation models and transfer learning have common characteristics. However, there are three key differences between computer vision models and LLMs:
- The amount of labour offset by automatable language tasks versus the labour offset by automatable vision tasks,
- The cost of acquiring LLM training data for specific tasks, and
- The computationally intensive nature of LLM prediction.
Investment in LLMs, computer vision models, and other prediction models most often require substantial tangible and intangible capital outlays. In resource allocation decisions, business and government leaders face competing uses of scarce production factors. Thus, understanding the economics of AI is necessary with the marginal costs and benefits of primary importance.
Uncertain spending estimates for a new and somewhat unknown technology can reduce projects to a sub-optimal level. While projects are delayed, the wait will be for AI-as-a-service firms to develop successful business models to deliver value to a very broad set of customers. Thus, expectations and feasts surrounding labour substitutability, improved worker productivity, and the aggregate macroeconomic benefits must be tempered by the guiding economics of AI.
Martin Fleming is Fellow, The Productivity Institute; Chief Revenue Scientist, Varicent; and Research Economist MIT FutureTech Project. He is the co-author of Beyond AI Exposure: Which Tasks are Cost-Effective to Automate with Computer Vision? by the Future Tech group at Massachusetts Institute of Technology.